Excessive staff workloads lie at the root of the current human resources crisis in health care. High workloads lead to fatigue, increase medical errors and contribute to burnout, absenteeism and staff such as nurses leaving the profession. We have been warned about these problems for years but little has been done to improve workload management in health care. With short-staffing apparently the norm, the costs for nurse absenteeism and overtime approach $1 billion each. This is not sustainable.
Simplistic efforts to reduce health-care costs by cutting budgets has heaped pressure on front-line nurses who have tried to make up the difference. After years of budget restrictions and associated workload increases, nurses and other front-line staff are at their breaking point and leaving the profession in droves. That the health-care system is hemorrhaging talent appears to be by design through the creation of unsustainable workloads. We need better workload management.
Fig. 1: The Better Work for Better Care Framework (explains how health-care system design choices determine workload levels for staff. These can affect nurses negatively in terms of fatigue which, in turn, has negative effects on patients (missed care in this case) with longer term worsening of outcomes and increases in system costs.
Efforts to shift to private for-profit health care will not help. Increasing shareholder value in health care can really be achieved only by increasing charges, adding unnecessary services and tests or by squeezing employees even more in search of “efficiencies.” This will only aggravate the excess workload problem. The U.S. for-profit health-care system costs are easily double those of most other countries – $12,318 per capita annually compared to Canada’s $5,905 (both figures in USD). At the same time, the U.S.’s for-profit system has some of the worst health outcomes in the world.
For-profit health care has proven itself to be a disaster. If anything, for-profit health care will make working conditions worse, the bleeding of staff will continue, and patients will suffer.
Regardless of the choice between expensive and ineffective for-profit care, or egalitarian public health care, there is a need to manage workload for the front line. Currently, the Canadian health-care system has no objective tools that can quantify nurses’ or personal support workers’ (PSW) workloads. The absence of such support tools explains why nurses might have 14 hours of work assigned for a 12-hour shift (Fig. 1).
Fig. 2: Simulation model results revealing excessive time demands placed on nurses in a 12-hour shift on a med-surg unit–- with and without breaks – based on institutional standard times and frequencies obtained from the GRASP system (From Neumann et al., 2023).
Workload management is complex and needs to go beyond merely the nurse:patient ratio approaches being discussed. In developing simulation tools that can quantify the time demands on nurses and impacts on patients, we have attempted to address critical workload contributors. This can include patient acuity and dependency levels; the design of the built environment; the assigned patient bed locations; the positioning of supplies and medications; the care routines and practice policies in place; and the infection control routine requirements. A one-size-fits-all patient assignment ignores individual patient acuity and dependency levels and may still leave some nurses overloaded and contribute to missed care for patients – a serious quality problem that threatens patient safety and increases mortality rates.
The modelling approaches we propose help account for changes in daily workload. They also \ help understand the impact of proposed changes in the system. Understanding how much care will be missed due to the extra data-entry work required with new technologies, such as electronic health records, is an important example. So is anticipating the impacts of outbreak or pandemic scenarios on both nurse workload and the amount of care that can be delivered.
By creating a virtual mirror of the care delivery process, the impact of each system design choice can be tested in isolation or in combination. The current status of the system can be analyzed for staff time utilization, walking distance measures, leftover care at the end of shift and the “waiting time” for a task (e.g., patient call) to be completed. These indicators can then be explored in any future configuration of the care unit that is required. Changing care task mixes, patient needs, work routines (e.g., nurse:patient ratios), repositioning of supplies and equipment – the impacts of all these can be quickly and precisely quantified. If, for example, teaching and emotional support are to be included in care practices, how does adding these tasks affect the shift-level workload?
Fig. 3: Schematic of the basic simulation model’s input and outputs used to track task time and performance patterns across whole shifts as specified in the inputs.
While models quantifying workloads are not a panacea, they can provide insights into the workload implications of current or planned operational scenarios on the time demands on nurses. There is no question about competency and the ability to adapt care to meet the individual care needs of the patient … provided there is enough time available to deliver that care. This is the current problem that needs evidence-informed decision-making from policy levels to the bedside.
Our presentations of this approach to professional nursing associations, labour organizations, national and provincial nursing leaders, practitioners and health-care system managers has been met with deep interest and support. This highlights the recognition of the need for evidence-informed workload management approaches in health care and broad support for this kind of objective workload measurement approach to help inform appropriate and safe staffing.
Our modelling work has shown that, during the COVID pandemic, nurses caring for COVID positive patients within their assignments actually had substantially lower biomechanical loading even as the time demands on them went up. This counterintuitive finding was driven by the need to don Personal Protective Equipment with each room entry. When the simulant nurse had all five assigned patients infected with COVID, half of the 12-hour shift was spent on infection control tasks that were less physically demanding than most care tasks. These measurements show how physical demands may decrease even as time demands and psychological pressures accumulate due to the increase in patient-care demands.
This scenario illustrates how modelling tools can be used to understand the complex drivers of workload. There remains a need to use such quantitative measures in practice. Evidence-based workload measures are a potential antidote to the antipathy that has met the long-standing and oft-repeated complaints and warnings made by the nursing profession. Policymakers and governments might wish to ignore the qualitative reports from these professionals since it becomes harder to maintain a “just do the job” stance when faced with objective evidence that the promised health-care services cannot be delivered with the available resources.
It’s not enough to simply tell staff “just add this data entry task to your routine” when those routines already include more hours of work than are available in a shift. This is health care, not button manufacturing; you can’t simply come back tomorrow and start the machines up again. This is classic “magical thinking” by policymakers.
Quantitative workload management is the antidote to magical thinking. It is unacceptable to think that simply asking nurses to work harder or work faster is appropriate workload management. The consequences of this approach are apparent in staff retirements, shortages and dissatisfaction. It is also apparent in the declines in quality of care.
The excess workload, and the associated loss of competencies, undermines efforts toward the provision of the fundamentals of care – person-centred care approaches that have shown better patient outcomes. Without consideration of all dimensions of patient care as part of workload, quality of care will remain elusive. Appropriate work standards, supported with evidence-informed workload quantification, is a way out of the “magical thinking” trap. Let’s start using it.
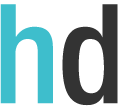
Very interesting approach and much needed. Thanks to both authors for outlining testing methodology and deferent aspects of workload measurements. Will be also interesting, in future, to expand the premise to nurses and allied health workers in community and home services as the existing gap is huge and widening between needs and outcomes.
Thank you Elias for your comment and interest. We are currently expanding the work in the complex continuing care context to understand personal support worker workload. We agree that there are opportunities to expand to other disciplines and practice sectors.